Big Data Analytics: What It Is and Why It is Important
Rohan Roy
Feb 21, 2022
Data Analytics






Data, as we know, comes in some form of characters, numbers, figures, statistics, etc. By processing or analyzing this data we get some meaningful information.
We have been storing and analyzing data since the modern era. Whether it is writing it down in a register or storing it in excel sheets.
What is Big Data?
Data that is so large, so fast or complex that is difficult to process using traditional methods is called Big data. Generally, big data is in Terabytes to Petabytes. There is no exact line between normal data and Big data.
Examples Of Big Data
Social Media generates 500+ Terabytes of new data every single day. Moreover, it's in the form of photos, videos, messages etc.
Airplane’s jet engine creates 10+terabytes of data within 30 minutes. Furthermore, with so many flights everyday, the data generated reaches Petabytes.

The V’s Of Big Data
Big data has the following characteristics:
Volume
Velocity
Variety
Variability
Value
(i) Volume – The volume of data determines whether or not it may be classified as Big Data. Furthermore, The Size of data plays a very important role. Therefore, when dealing with Big Data, it is vital to consider 'Volume.'
(ii) Velocity – Velocity here means how fast the data is generated. The real potential of the data is determined by how fast it is processed and generated. The data flows from different sources like social media, smart phones, machines, etc. The flow of data is continuous and massive.
(iii) Variety – Variety here means different nature and sources of data. They can be structured, semi structured or unstructured. Previously, spreadsheets and databases were the only data sources. However, today, data comes in the form of photos, videos, audio, emails, PDFs, etc. These are also being considered in the analysis.
Structured data: properly defined and organized. Example an excel sheet.
Semi Structured data: Partially organized data. Example a google search
Unstructured data: Unorganized data which cannot be arranged in traditional rows and columns. Example photos, videos, audios etc.
(iv) Variability – Variability refers to the inconsistencies that data can display at times. Moreover, the data gets messy and quality and accuracy are difficult to control. Thus, limiting the ability to effectively handle and manage data.
(v)Value - After the 4 V’s, there comes one more V which stands for Value.The data with no value is useless. It should be converted to something meaningful.Data must be turned into something useful in order to extract information or its of no relevance.
What is Big Data Analytics?
The use of advanced analytic techniques to very large, heterogeneous data sets is known as big data analytics.Furthermore, that include structured, semi-structured and unstructured data, iin different sizes from terabytes to petabytes, and from different sources.
Big data analytics is the process of analysing massive amounts of data to find correlations, hidden patterns, and other insights. We can analyse the data much faster as compared to the traditional methods and can get answers almost immediately. Machine learning, text analytics, data mining, statistics, predictive analytics, and natural language processing are examples of big data analytics.These approaches can be used individually or in conjunction with existing business data to get new insights from previously untapped data sources.
Most firms now realise that if they capture all of the data that flows into their systems, they can use Big Data analytics to extract great value. Businesses were employing basic analytics (just figures on a spreadsheet that were manually inspected) to identify insights and trends as early as the 1950s, decades before the term "big data" was coined.
The majority of businesses have a large amount of data. Many people recognise the importance of harnessing data and extracting value from it. But how do you do it? The newest thinking on the convergence of big data and analytics is covered in these resources.
Why is Big Data Analytics Important?
Big data analytics assists businesses in utilizing their data and identifying new opportunities. As a result, smarter business decisions, more effective operations, higher profits, and happier consumers. Below are some advantages of it:
Cost reduction. When it comes to storing large amounts of data Big Data tools such as Hadoop and Cloud based analytics can reduce significant amounts of cost. In addition to that they can identify more efficient ways of doing business.
Faster, better decision making. Businesses can evaluate information instantaneously – and make decisions based on what they've learned – thanks to the speed of Hadoop and in-memory analytics, as well as the ability to study new sources of data.
New products and services. With such amazing insights through analytics, companies can understand customers' needs. Therefore, with big data analytics, businesses can create new products to meet customers needs.
Businesses can use external intelligence to make decisions. They can step ahead in strategies with access to data from search engines and social media.
Improved customer service. Traditional consumer feedback systems are being replaced by Big Data tools. Consumer responses are analyzed and evaluated using Big Data Analytics Services and natural language processing technology in these new platforms.
Early identification of risk to the product/services, if any
Better operational efficiency
Big Data Analytics Tools and Technology
Big data analytics is too broad to be summed up in a single tool or technology. Instead, a combination of tools is used to collect, process, cleanse, and analyze large amounts of data. The following is a list of some of the most important tools in big data analytics.
Hadoop is an open-source framework for storing and processing large datasets on commodity hardware clusters. This framework is open-source and capable of handling massive amounts of organized and unstructured data, making it an essential component of any big data project.
Spark is an open source cluster computing framework that provides an interface for programming complete clusters by utilizing implicit data parallelism and fault tolerance. For quick computing, Spark can handle batch and stream processing.
MapReduce is an essential part of the Hadoop framework that serves two purposes. The first is mapping, which filters data and distributes it among cluster nodes. The second method is reduction, which organizes and condenses the results from each node in order to respond to a query.
YARN stands for “Yet Another Resource Negotiator.” It's a component of Hadoop's second generation. Job scheduling and resource management in the cluster are aided by cluster management technology.
NoSQL databases are non-relational data management systems that don't require a set schema, making them an excellent choice for large amounts of unstructured data. The term "not only SQL" refers to the fact that these databases can handle a wide range of data models.
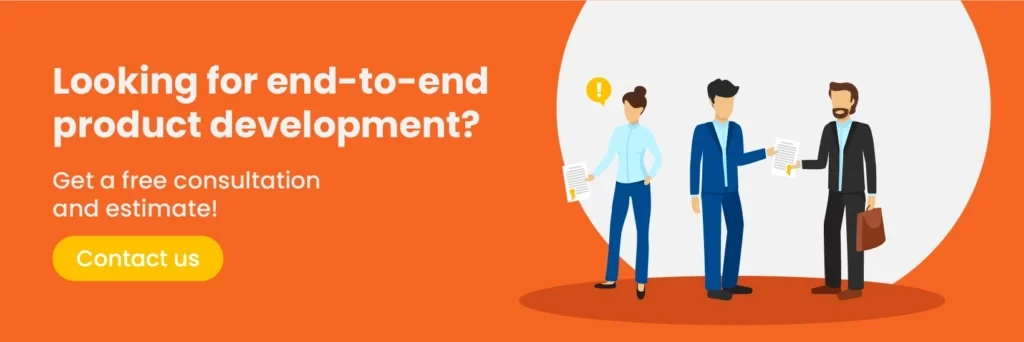
Data, as we know, comes in some form of characters, numbers, figures, statistics, etc. By processing or analyzing this data we get some meaningful information.
We have been storing and analyzing data since the modern era. Whether it is writing it down in a register or storing it in excel sheets.
What is Big Data?
Data that is so large, so fast or complex that is difficult to process using traditional methods is called Big data. Generally, big data is in Terabytes to Petabytes. There is no exact line between normal data and Big data.
Examples Of Big Data
Social Media generates 500+ Terabytes of new data every single day. Moreover, it's in the form of photos, videos, messages etc.
Airplane’s jet engine creates 10+terabytes of data within 30 minutes. Furthermore, with so many flights everyday, the data generated reaches Petabytes.

The V’s Of Big Data
Big data has the following characteristics:
Volume
Velocity
Variety
Variability
Value
(i) Volume – The volume of data determines whether or not it may be classified as Big Data. Furthermore, The Size of data plays a very important role. Therefore, when dealing with Big Data, it is vital to consider 'Volume.'
(ii) Velocity – Velocity here means how fast the data is generated. The real potential of the data is determined by how fast it is processed and generated. The data flows from different sources like social media, smart phones, machines, etc. The flow of data is continuous and massive.
(iii) Variety – Variety here means different nature and sources of data. They can be structured, semi structured or unstructured. Previously, spreadsheets and databases were the only data sources. However, today, data comes in the form of photos, videos, audio, emails, PDFs, etc. These are also being considered in the analysis.
Structured data: properly defined and organized. Example an excel sheet.
Semi Structured data: Partially organized data. Example a google search
Unstructured data: Unorganized data which cannot be arranged in traditional rows and columns. Example photos, videos, audios etc.
(iv) Variability – Variability refers to the inconsistencies that data can display at times. Moreover, the data gets messy and quality and accuracy are difficult to control. Thus, limiting the ability to effectively handle and manage data.
(v)Value - After the 4 V’s, there comes one more V which stands for Value.The data with no value is useless. It should be converted to something meaningful.Data must be turned into something useful in order to extract information or its of no relevance.
What is Big Data Analytics?
The use of advanced analytic techniques to very large, heterogeneous data sets is known as big data analytics.Furthermore, that include structured, semi-structured and unstructured data, iin different sizes from terabytes to petabytes, and from different sources.
Big data analytics is the process of analysing massive amounts of data to find correlations, hidden patterns, and other insights. We can analyse the data much faster as compared to the traditional methods and can get answers almost immediately. Machine learning, text analytics, data mining, statistics, predictive analytics, and natural language processing are examples of big data analytics.These approaches can be used individually or in conjunction with existing business data to get new insights from previously untapped data sources.
Most firms now realise that if they capture all of the data that flows into their systems, they can use Big Data analytics to extract great value. Businesses were employing basic analytics (just figures on a spreadsheet that were manually inspected) to identify insights and trends as early as the 1950s, decades before the term "big data" was coined.
The majority of businesses have a large amount of data. Many people recognise the importance of harnessing data and extracting value from it. But how do you do it? The newest thinking on the convergence of big data and analytics is covered in these resources.
Why is Big Data Analytics Important?
Big data analytics assists businesses in utilizing their data and identifying new opportunities. As a result, smarter business decisions, more effective operations, higher profits, and happier consumers. Below are some advantages of it:
Cost reduction. When it comes to storing large amounts of data Big Data tools such as Hadoop and Cloud based analytics can reduce significant amounts of cost. In addition to that they can identify more efficient ways of doing business.
Faster, better decision making. Businesses can evaluate information instantaneously – and make decisions based on what they've learned – thanks to the speed of Hadoop and in-memory analytics, as well as the ability to study new sources of data.
New products and services. With such amazing insights through analytics, companies can understand customers' needs. Therefore, with big data analytics, businesses can create new products to meet customers needs.
Businesses can use external intelligence to make decisions. They can step ahead in strategies with access to data from search engines and social media.
Improved customer service. Traditional consumer feedback systems are being replaced by Big Data tools. Consumer responses are analyzed and evaluated using Big Data Analytics Services and natural language processing technology in these new platforms.
Early identification of risk to the product/services, if any
Better operational efficiency
Big Data Analytics Tools and Technology
Big data analytics is too broad to be summed up in a single tool or technology. Instead, a combination of tools is used to collect, process, cleanse, and analyze large amounts of data. The following is a list of some of the most important tools in big data analytics.
Hadoop is an open-source framework for storing and processing large datasets on commodity hardware clusters. This framework is open-source and capable of handling massive amounts of organized and unstructured data, making it an essential component of any big data project.
Spark is an open source cluster computing framework that provides an interface for programming complete clusters by utilizing implicit data parallelism and fault tolerance. For quick computing, Spark can handle batch and stream processing.
MapReduce is an essential part of the Hadoop framework that serves two purposes. The first is mapping, which filters data and distributes it among cluster nodes. The second method is reduction, which organizes and condenses the results from each node in order to respond to a query.
YARN stands for “Yet Another Resource Negotiator.” It's a component of Hadoop's second generation. Job scheduling and resource management in the cluster are aided by cluster management technology.
NoSQL databases are non-relational data management systems that don't require a set schema, making them an excellent choice for large amounts of unstructured data. The term "not only SQL" refers to the fact that these databases can handle a wide range of data models.
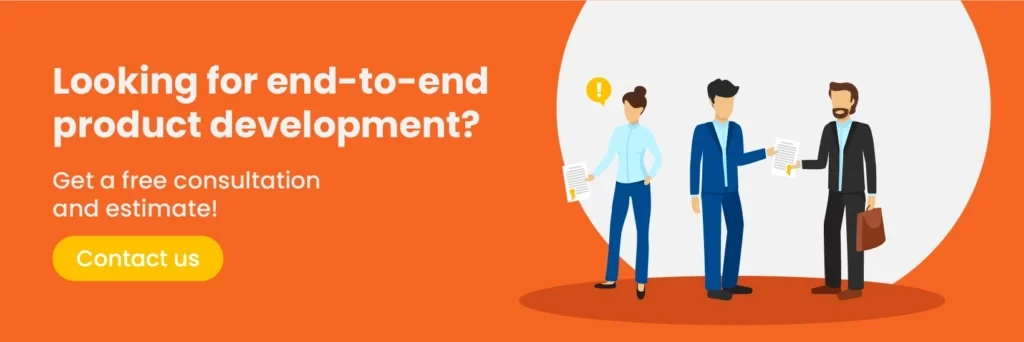
Data, as we know, comes in some form of characters, numbers, figures, statistics, etc. By processing or analyzing this data we get some meaningful information.
We have been storing and analyzing data since the modern era. Whether it is writing it down in a register or storing it in excel sheets.
What is Big Data?
Data that is so large, so fast or complex that is difficult to process using traditional methods is called Big data. Generally, big data is in Terabytes to Petabytes. There is no exact line between normal data and Big data.
Examples Of Big Data
Social Media generates 500+ Terabytes of new data every single day. Moreover, it's in the form of photos, videos, messages etc.
Airplane’s jet engine creates 10+terabytes of data within 30 minutes. Furthermore, with so many flights everyday, the data generated reaches Petabytes.

The V’s Of Big Data
Big data has the following characteristics:
Volume
Velocity
Variety
Variability
Value
(i) Volume – The volume of data determines whether or not it may be classified as Big Data. Furthermore, The Size of data plays a very important role. Therefore, when dealing with Big Data, it is vital to consider 'Volume.'
(ii) Velocity – Velocity here means how fast the data is generated. The real potential of the data is determined by how fast it is processed and generated. The data flows from different sources like social media, smart phones, machines, etc. The flow of data is continuous and massive.
(iii) Variety – Variety here means different nature and sources of data. They can be structured, semi structured or unstructured. Previously, spreadsheets and databases were the only data sources. However, today, data comes in the form of photos, videos, audio, emails, PDFs, etc. These are also being considered in the analysis.
Structured data: properly defined and organized. Example an excel sheet.
Semi Structured data: Partially organized data. Example a google search
Unstructured data: Unorganized data which cannot be arranged in traditional rows and columns. Example photos, videos, audios etc.
(iv) Variability – Variability refers to the inconsistencies that data can display at times. Moreover, the data gets messy and quality and accuracy are difficult to control. Thus, limiting the ability to effectively handle and manage data.
(v)Value - After the 4 V’s, there comes one more V which stands for Value.The data with no value is useless. It should be converted to something meaningful.Data must be turned into something useful in order to extract information or its of no relevance.
What is Big Data Analytics?
The use of advanced analytic techniques to very large, heterogeneous data sets is known as big data analytics.Furthermore, that include structured, semi-structured and unstructured data, iin different sizes from terabytes to petabytes, and from different sources.
Big data analytics is the process of analysing massive amounts of data to find correlations, hidden patterns, and other insights. We can analyse the data much faster as compared to the traditional methods and can get answers almost immediately. Machine learning, text analytics, data mining, statistics, predictive analytics, and natural language processing are examples of big data analytics.These approaches can be used individually or in conjunction with existing business data to get new insights from previously untapped data sources.
Most firms now realise that if they capture all of the data that flows into their systems, they can use Big Data analytics to extract great value. Businesses were employing basic analytics (just figures on a spreadsheet that were manually inspected) to identify insights and trends as early as the 1950s, decades before the term "big data" was coined.
The majority of businesses have a large amount of data. Many people recognise the importance of harnessing data and extracting value from it. But how do you do it? The newest thinking on the convergence of big data and analytics is covered in these resources.
Why is Big Data Analytics Important?
Big data analytics assists businesses in utilizing their data and identifying new opportunities. As a result, smarter business decisions, more effective operations, higher profits, and happier consumers. Below are some advantages of it:
Cost reduction. When it comes to storing large amounts of data Big Data tools such as Hadoop and Cloud based analytics can reduce significant amounts of cost. In addition to that they can identify more efficient ways of doing business.
Faster, better decision making. Businesses can evaluate information instantaneously – and make decisions based on what they've learned – thanks to the speed of Hadoop and in-memory analytics, as well as the ability to study new sources of data.
New products and services. With such amazing insights through analytics, companies can understand customers' needs. Therefore, with big data analytics, businesses can create new products to meet customers needs.
Businesses can use external intelligence to make decisions. They can step ahead in strategies with access to data from search engines and social media.
Improved customer service. Traditional consumer feedback systems are being replaced by Big Data tools. Consumer responses are analyzed and evaluated using Big Data Analytics Services and natural language processing technology in these new platforms.
Early identification of risk to the product/services, if any
Better operational efficiency
Big Data Analytics Tools and Technology
Big data analytics is too broad to be summed up in a single tool or technology. Instead, a combination of tools is used to collect, process, cleanse, and analyze large amounts of data. The following is a list of some of the most important tools in big data analytics.
Hadoop is an open-source framework for storing and processing large datasets on commodity hardware clusters. This framework is open-source and capable of handling massive amounts of organized and unstructured data, making it an essential component of any big data project.
Spark is an open source cluster computing framework that provides an interface for programming complete clusters by utilizing implicit data parallelism and fault tolerance. For quick computing, Spark can handle batch and stream processing.
MapReduce is an essential part of the Hadoop framework that serves two purposes. The first is mapping, which filters data and distributes it among cluster nodes. The second method is reduction, which organizes and condenses the results from each node in order to respond to a query.
YARN stands for “Yet Another Resource Negotiator.” It's a component of Hadoop's second generation. Job scheduling and resource management in the cluster are aided by cluster management technology.
NoSQL databases are non-relational data management systems that don't require a set schema, making them an excellent choice for large amounts of unstructured data. The term "not only SQL" refers to the fact that these databases can handle a wide range of data models.
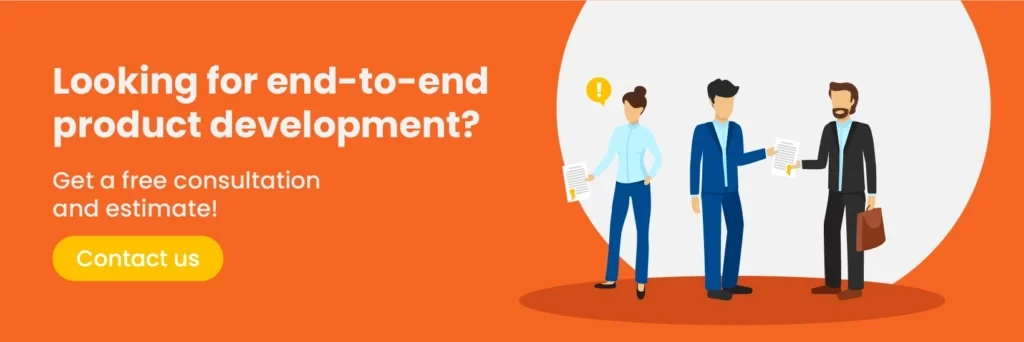
Data, as we know, comes in some form of characters, numbers, figures, statistics, etc. By processing or analyzing this data we get some meaningful information.
We have been storing and analyzing data since the modern era. Whether it is writing it down in a register or storing it in excel sheets.
What is Big Data?
Data that is so large, so fast or complex that is difficult to process using traditional methods is called Big data. Generally, big data is in Terabytes to Petabytes. There is no exact line between normal data and Big data.
Examples Of Big Data
Social Media generates 500+ Terabytes of new data every single day. Moreover, it's in the form of photos, videos, messages etc.
Airplane’s jet engine creates 10+terabytes of data within 30 minutes. Furthermore, with so many flights everyday, the data generated reaches Petabytes.

The V’s Of Big Data
Big data has the following characteristics:
Volume
Velocity
Variety
Variability
Value
(i) Volume – The volume of data determines whether or not it may be classified as Big Data. Furthermore, The Size of data plays a very important role. Therefore, when dealing with Big Data, it is vital to consider 'Volume.'
(ii) Velocity – Velocity here means how fast the data is generated. The real potential of the data is determined by how fast it is processed and generated. The data flows from different sources like social media, smart phones, machines, etc. The flow of data is continuous and massive.
(iii) Variety – Variety here means different nature and sources of data. They can be structured, semi structured or unstructured. Previously, spreadsheets and databases were the only data sources. However, today, data comes in the form of photos, videos, audio, emails, PDFs, etc. These are also being considered in the analysis.
Structured data: properly defined and organized. Example an excel sheet.
Semi Structured data: Partially organized data. Example a google search
Unstructured data: Unorganized data which cannot be arranged in traditional rows and columns. Example photos, videos, audios etc.
(iv) Variability – Variability refers to the inconsistencies that data can display at times. Moreover, the data gets messy and quality and accuracy are difficult to control. Thus, limiting the ability to effectively handle and manage data.
(v)Value - After the 4 V’s, there comes one more V which stands for Value.The data with no value is useless. It should be converted to something meaningful.Data must be turned into something useful in order to extract information or its of no relevance.
What is Big Data Analytics?
The use of advanced analytic techniques to very large, heterogeneous data sets is known as big data analytics.Furthermore, that include structured, semi-structured and unstructured data, iin different sizes from terabytes to petabytes, and from different sources.
Big data analytics is the process of analysing massive amounts of data to find correlations, hidden patterns, and other insights. We can analyse the data much faster as compared to the traditional methods and can get answers almost immediately. Machine learning, text analytics, data mining, statistics, predictive analytics, and natural language processing are examples of big data analytics.These approaches can be used individually or in conjunction with existing business data to get new insights from previously untapped data sources.
Most firms now realise that if they capture all of the data that flows into their systems, they can use Big Data analytics to extract great value. Businesses were employing basic analytics (just figures on a spreadsheet that were manually inspected) to identify insights and trends as early as the 1950s, decades before the term "big data" was coined.
The majority of businesses have a large amount of data. Many people recognise the importance of harnessing data and extracting value from it. But how do you do it? The newest thinking on the convergence of big data and analytics is covered in these resources.
Why is Big Data Analytics Important?
Big data analytics assists businesses in utilizing their data and identifying new opportunities. As a result, smarter business decisions, more effective operations, higher profits, and happier consumers. Below are some advantages of it:
Cost reduction. When it comes to storing large amounts of data Big Data tools such as Hadoop and Cloud based analytics can reduce significant amounts of cost. In addition to that they can identify more efficient ways of doing business.
Faster, better decision making. Businesses can evaluate information instantaneously – and make decisions based on what they've learned – thanks to the speed of Hadoop and in-memory analytics, as well as the ability to study new sources of data.
New products and services. With such amazing insights through analytics, companies can understand customers' needs. Therefore, with big data analytics, businesses can create new products to meet customers needs.
Businesses can use external intelligence to make decisions. They can step ahead in strategies with access to data from search engines and social media.
Improved customer service. Traditional consumer feedback systems are being replaced by Big Data tools. Consumer responses are analyzed and evaluated using Big Data Analytics Services and natural language processing technology in these new platforms.
Early identification of risk to the product/services, if any
Better operational efficiency
Big Data Analytics Tools and Technology
Big data analytics is too broad to be summed up in a single tool or technology. Instead, a combination of tools is used to collect, process, cleanse, and analyze large amounts of data. The following is a list of some of the most important tools in big data analytics.
Hadoop is an open-source framework for storing and processing large datasets on commodity hardware clusters. This framework is open-source and capable of handling massive amounts of organized and unstructured data, making it an essential component of any big data project.
Spark is an open source cluster computing framework that provides an interface for programming complete clusters by utilizing implicit data parallelism and fault tolerance. For quick computing, Spark can handle batch and stream processing.
MapReduce is an essential part of the Hadoop framework that serves two purposes. The first is mapping, which filters data and distributes it among cluster nodes. The second method is reduction, which organizes and condenses the results from each node in order to respond to a query.
YARN stands for “Yet Another Resource Negotiator.” It's a component of Hadoop's second generation. Job scheduling and resource management in the cluster are aided by cluster management technology.
NoSQL databases are non-relational data management systems that don't require a set schema, making them an excellent choice for large amounts of unstructured data. The term "not only SQL" refers to the fact that these databases can handle a wide range of data models.
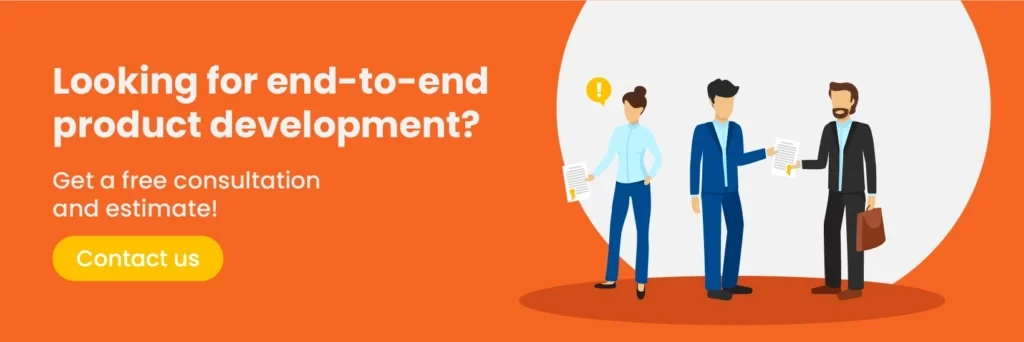
Data, as we know, comes in some form of characters, numbers, figures, statistics, etc. By processing or analyzing this data we get some meaningful information.
We have been storing and analyzing data since the modern era. Whether it is writing it down in a register or storing it in excel sheets.
What is Big Data?
Data that is so large, so fast or complex that is difficult to process using traditional methods is called Big data. Generally, big data is in Terabytes to Petabytes. There is no exact line between normal data and Big data.
Examples Of Big Data
Social Media generates 500+ Terabytes of new data every single day. Moreover, it's in the form of photos, videos, messages etc.
Airplane’s jet engine creates 10+terabytes of data within 30 minutes. Furthermore, with so many flights everyday, the data generated reaches Petabytes.

The V’s Of Big Data
Big data has the following characteristics:
Volume
Velocity
Variety
Variability
Value
(i) Volume – The volume of data determines whether or not it may be classified as Big Data. Furthermore, The Size of data plays a very important role. Therefore, when dealing with Big Data, it is vital to consider 'Volume.'
(ii) Velocity – Velocity here means how fast the data is generated. The real potential of the data is determined by how fast it is processed and generated. The data flows from different sources like social media, smart phones, machines, etc. The flow of data is continuous and massive.
(iii) Variety – Variety here means different nature and sources of data. They can be structured, semi structured or unstructured. Previously, spreadsheets and databases were the only data sources. However, today, data comes in the form of photos, videos, audio, emails, PDFs, etc. These are also being considered in the analysis.
Structured data: properly defined and organized. Example an excel sheet.
Semi Structured data: Partially organized data. Example a google search
Unstructured data: Unorganized data which cannot be arranged in traditional rows and columns. Example photos, videos, audios etc.
(iv) Variability – Variability refers to the inconsistencies that data can display at times. Moreover, the data gets messy and quality and accuracy are difficult to control. Thus, limiting the ability to effectively handle and manage data.
(v)Value - After the 4 V’s, there comes one more V which stands for Value.The data with no value is useless. It should be converted to something meaningful.Data must be turned into something useful in order to extract information or its of no relevance.
What is Big Data Analytics?
The use of advanced analytic techniques to very large, heterogeneous data sets is known as big data analytics.Furthermore, that include structured, semi-structured and unstructured data, iin different sizes from terabytes to petabytes, and from different sources.
Big data analytics is the process of analysing massive amounts of data to find correlations, hidden patterns, and other insights. We can analyse the data much faster as compared to the traditional methods and can get answers almost immediately. Machine learning, text analytics, data mining, statistics, predictive analytics, and natural language processing are examples of big data analytics.These approaches can be used individually or in conjunction with existing business data to get new insights from previously untapped data sources.
Most firms now realise that if they capture all of the data that flows into their systems, they can use Big Data analytics to extract great value. Businesses were employing basic analytics (just figures on a spreadsheet that were manually inspected) to identify insights and trends as early as the 1950s, decades before the term "big data" was coined.
The majority of businesses have a large amount of data. Many people recognise the importance of harnessing data and extracting value from it. But how do you do it? The newest thinking on the convergence of big data and analytics is covered in these resources.
Why is Big Data Analytics Important?
Big data analytics assists businesses in utilizing their data and identifying new opportunities. As a result, smarter business decisions, more effective operations, higher profits, and happier consumers. Below are some advantages of it:
Cost reduction. When it comes to storing large amounts of data Big Data tools such as Hadoop and Cloud based analytics can reduce significant amounts of cost. In addition to that they can identify more efficient ways of doing business.
Faster, better decision making. Businesses can evaluate information instantaneously – and make decisions based on what they've learned – thanks to the speed of Hadoop and in-memory analytics, as well as the ability to study new sources of data.
New products and services. With such amazing insights through analytics, companies can understand customers' needs. Therefore, with big data analytics, businesses can create new products to meet customers needs.
Businesses can use external intelligence to make decisions. They can step ahead in strategies with access to data from search engines and social media.
Improved customer service. Traditional consumer feedback systems are being replaced by Big Data tools. Consumer responses are analyzed and evaluated using Big Data Analytics Services and natural language processing technology in these new platforms.
Early identification of risk to the product/services, if any
Better operational efficiency
Big Data Analytics Tools and Technology
Big data analytics is too broad to be summed up in a single tool or technology. Instead, a combination of tools is used to collect, process, cleanse, and analyze large amounts of data. The following is a list of some of the most important tools in big data analytics.
Hadoop is an open-source framework for storing and processing large datasets on commodity hardware clusters. This framework is open-source and capable of handling massive amounts of organized and unstructured data, making it an essential component of any big data project.
Spark is an open source cluster computing framework that provides an interface for programming complete clusters by utilizing implicit data parallelism and fault tolerance. For quick computing, Spark can handle batch and stream processing.
MapReduce is an essential part of the Hadoop framework that serves two purposes. The first is mapping, which filters data and distributes it among cluster nodes. The second method is reduction, which organizes and condenses the results from each node in order to respond to a query.
YARN stands for “Yet Another Resource Negotiator.” It's a component of Hadoop's second generation. Job scheduling and resource management in the cluster are aided by cluster management technology.
NoSQL databases are non-relational data management systems that don't require a set schema, making them an excellent choice for large amounts of unstructured data. The term "not only SQL" refers to the fact that these databases can handle a wide range of data models.
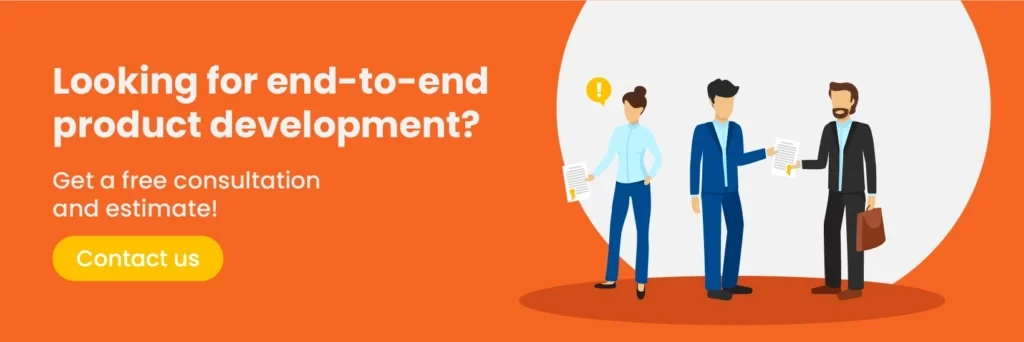
Data, as we know, comes in some form of characters, numbers, figures, statistics, etc. By processing or analyzing this data we get some meaningful information.
We have been storing and analyzing data since the modern era. Whether it is writing it down in a register or storing it in excel sheets.
What is Big Data?
Data that is so large, so fast or complex that is difficult to process using traditional methods is called Big data. Generally, big data is in Terabytes to Petabytes. There is no exact line between normal data and Big data.
Examples Of Big Data
Social Media generates 500+ Terabytes of new data every single day. Moreover, it's in the form of photos, videos, messages etc.
Airplane’s jet engine creates 10+terabytes of data within 30 minutes. Furthermore, with so many flights everyday, the data generated reaches Petabytes.

The V’s Of Big Data
Big data has the following characteristics:
Volume
Velocity
Variety
Variability
Value
(i) Volume – The volume of data determines whether or not it may be classified as Big Data. Furthermore, The Size of data plays a very important role. Therefore, when dealing with Big Data, it is vital to consider 'Volume.'
(ii) Velocity – Velocity here means how fast the data is generated. The real potential of the data is determined by how fast it is processed and generated. The data flows from different sources like social media, smart phones, machines, etc. The flow of data is continuous and massive.
(iii) Variety – Variety here means different nature and sources of data. They can be structured, semi structured or unstructured. Previously, spreadsheets and databases were the only data sources. However, today, data comes in the form of photos, videos, audio, emails, PDFs, etc. These are also being considered in the analysis.
Structured data: properly defined and organized. Example an excel sheet.
Semi Structured data: Partially organized data. Example a google search
Unstructured data: Unorganized data which cannot be arranged in traditional rows and columns. Example photos, videos, audios etc.
(iv) Variability – Variability refers to the inconsistencies that data can display at times. Moreover, the data gets messy and quality and accuracy are difficult to control. Thus, limiting the ability to effectively handle and manage data.
(v)Value - After the 4 V’s, there comes one more V which stands for Value.The data with no value is useless. It should be converted to something meaningful.Data must be turned into something useful in order to extract information or its of no relevance.
What is Big Data Analytics?
The use of advanced analytic techniques to very large, heterogeneous data sets is known as big data analytics.Furthermore, that include structured, semi-structured and unstructured data, iin different sizes from terabytes to petabytes, and from different sources.
Big data analytics is the process of analysing massive amounts of data to find correlations, hidden patterns, and other insights. We can analyse the data much faster as compared to the traditional methods and can get answers almost immediately. Machine learning, text analytics, data mining, statistics, predictive analytics, and natural language processing are examples of big data analytics.These approaches can be used individually or in conjunction with existing business data to get new insights from previously untapped data sources.
Most firms now realise that if they capture all of the data that flows into their systems, they can use Big Data analytics to extract great value. Businesses were employing basic analytics (just figures on a spreadsheet that were manually inspected) to identify insights and trends as early as the 1950s, decades before the term "big data" was coined.
The majority of businesses have a large amount of data. Many people recognise the importance of harnessing data and extracting value from it. But how do you do it? The newest thinking on the convergence of big data and analytics is covered in these resources.
Why is Big Data Analytics Important?
Big data analytics assists businesses in utilizing their data and identifying new opportunities. As a result, smarter business decisions, more effective operations, higher profits, and happier consumers. Below are some advantages of it:
Cost reduction. When it comes to storing large amounts of data Big Data tools such as Hadoop and Cloud based analytics can reduce significant amounts of cost. In addition to that they can identify more efficient ways of doing business.
Faster, better decision making. Businesses can evaluate information instantaneously – and make decisions based on what they've learned – thanks to the speed of Hadoop and in-memory analytics, as well as the ability to study new sources of data.
New products and services. With such amazing insights through analytics, companies can understand customers' needs. Therefore, with big data analytics, businesses can create new products to meet customers needs.
Businesses can use external intelligence to make decisions. They can step ahead in strategies with access to data from search engines and social media.
Improved customer service. Traditional consumer feedback systems are being replaced by Big Data tools. Consumer responses are analyzed and evaluated using Big Data Analytics Services and natural language processing technology in these new platforms.
Early identification of risk to the product/services, if any
Better operational efficiency
Big Data Analytics Tools and Technology
Big data analytics is too broad to be summed up in a single tool or technology. Instead, a combination of tools is used to collect, process, cleanse, and analyze large amounts of data. The following is a list of some of the most important tools in big data analytics.
Hadoop is an open-source framework for storing and processing large datasets on commodity hardware clusters. This framework is open-source and capable of handling massive amounts of organized and unstructured data, making it an essential component of any big data project.
Spark is an open source cluster computing framework that provides an interface for programming complete clusters by utilizing implicit data parallelism and fault tolerance. For quick computing, Spark can handle batch and stream processing.
MapReduce is an essential part of the Hadoop framework that serves two purposes. The first is mapping, which filters data and distributes it among cluster nodes. The second method is reduction, which organizes and condenses the results from each node in order to respond to a query.
YARN stands for “Yet Another Resource Negotiator.” It's a component of Hadoop's second generation. Job scheduling and resource management in the cluster are aided by cluster management technology.
NoSQL databases are non-relational data management systems that don't require a set schema, making them an excellent choice for large amounts of unstructured data. The term "not only SQL" refers to the fact that these databases can handle a wide range of data models.
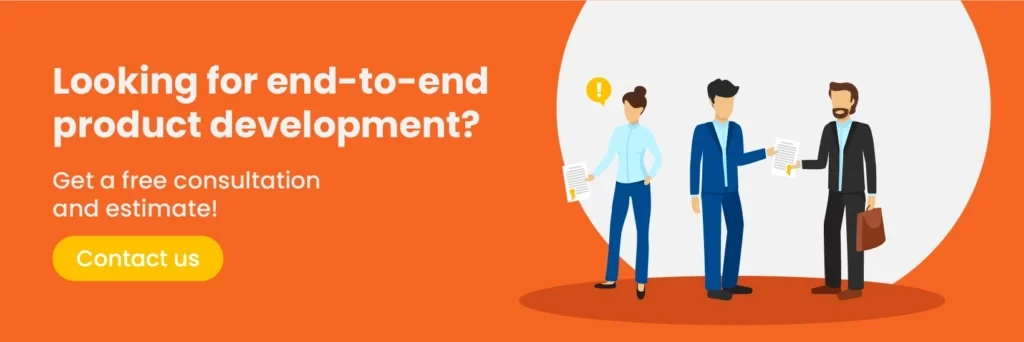
Recent Posts
Transform your vision into reality with Custom Software Development
Get Started
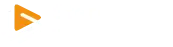
Office Address:
743A, Gera’s Imperium Rise,Hinjewadi Phase II, Rajiv Gandhi Infotech Park, Near Wipro Circle, Pune- 411057, Maharashtra, India
Ai
Services
Technologies
Transform your vision into reality with Custom Software Development
Get Started
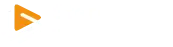
Office Address:
743A, Gera’s Imperium Rise,Hinjewadi Phase II, Rajiv Gandhi Infotech Park, Near Wipro Circle, Pune- 411057, Maharashtra, India
Ai
Services
Technologies
Transform your vision into reality with Custom Software Development
Get Started
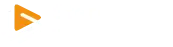
Office Address:
743A, Gera’s Imperium Rise,Hinjewadi Phase II, Rajiv Gandhi Infotech Park, Near Wipro Circle, Pune- 411057, Maharashtra, India
Technologies
Transform your vision into reality with Custom Software Development
Get Started
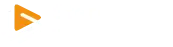
Office Address:
743A, Gera’s Imperium Rise,Hinjewadi Phase II, Rajiv Gandhi Infotech Park, Near Wipro Circle, Pune- 411057, Maharashtra, India
Ai
Services
Technologies
Transform your vision into reality with Custom Software Development
Get Started
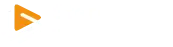
Office Address:
743A, Gera’s Imperium Rise,Hinjewadi Phase II, Rajiv Gandhi Infotech Park, Near Wipro Circle, Pune- 411057, Maharashtra, India
Ai
Services
Technologies
Transform your vision into reality with Custom Software Development
Get Started
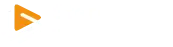
Office Address:
743A, Gera’s Imperium Rise,Hinjewadi Phase II, Rajiv Gandhi Infotech Park, Near Wipro Circle, Pune- 411057, Maharashtra, India